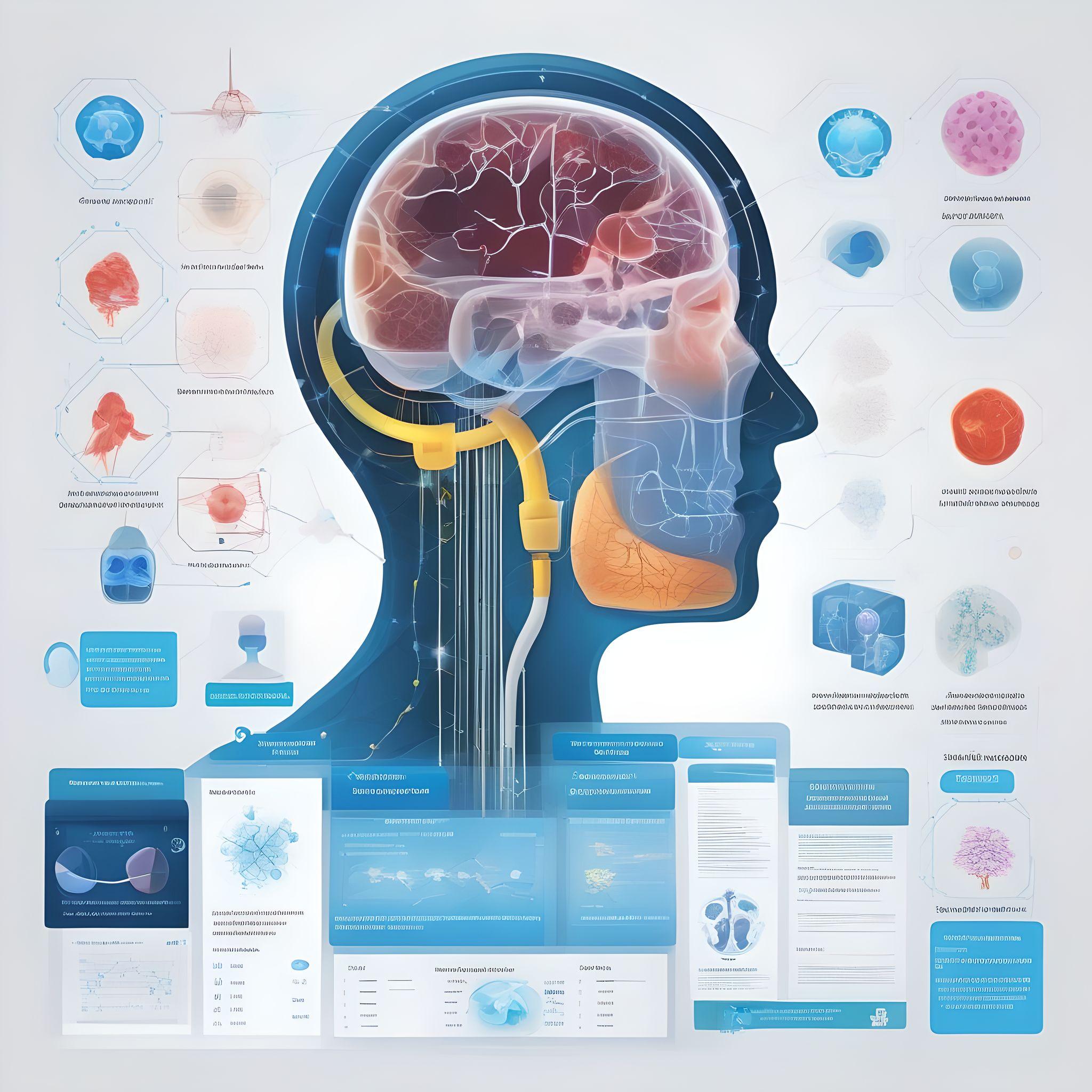
Healing with Algorithms: Generative AI in HealthCare
Generative AI is a type of artificial intelligence capable of creating new data, such as text, images, and even audio. By learning from existing data, it generates entirely new content. It is fascinating to observe how AI has revolutionized healthcare. Among the generative AI models, diffusion models and transformer-based models stand out as leading examples. Transformer-based models have been used for protein structure prediction, clinical documentation and information extraction, diagnostic assistance, medical imaging and radiology interpretation, clinical decision support, medical coding and billing, and drug design and molecular representation, while diffusion models can do tasks like image reconstruction, image to image translation, image generation, image classification, and other applications. Imagine a world where artificial intelligence can enhance the clarity of your MRI scans, translate CT images into detailed MRI-like visuals, and even predict the most effective treatments based on your unique health profile. This isn't science fiction—it's happening now. Dive into this transformative journey as we explore how cutting-edge AI technologies are not only improving diagnostic accuracy but also providing personalized health support through intelligent chatbots. Let’s explore the applications of Generative AI in healthcare.
Generative AI enhances the quality of medical images
Medical images often suffer from noise due to various factors like low radiation doses or fast imaging techniques. Generative AI models, particularly Generative Adversarial Networks (GANs), are trained to distinguish between noise and true anatomical structures. By learning the statistical properties of noise, these models can effectively filter it out, resulting in clearer images.
Modality Translation
Generative AI can translate images from one modality to another (e.g., converting a CT scan into an MRI-like image). This is beneficial because different imaging modalities provide complementary information. By combining these, clinicians can gain a more comprehensive view of the patient's condition.
Resolution Enhancement
Generative AI can improve the resolution of medical images by using techniques such as super-resolution. These models are trained on high-resolution and low-resolution image pairs, learning to generate high-resolution details from low-resolution inputs. This process enhances the visibility of fine anatomical details, which is crucial for accurate diagnosis and treatment planning.
Image Synthesis
AI models can generate synthetic medical images that mimic the properties of real images. This can be useful in creating datasets for training other AI models or for educational purposes. Synthetic images help in augmenting datasets, especially when real images are scarce
AI Chatbot in Healthcare
We can also design a chatbot for personalized health support using generative AI. It requires integration with electronic health records to access patient data securely. Natural language understanding is essential for accurately interpreting patient queries and emotional nuances. Sentiment analysis enables the chatbot to respond empathetically, while machine learning algorithms customize advice based on each person's health profile. Continuous learning mechanisms refine the bot's responses over time, enhancing accuracy and relevance. Strict security measures are crucial to ensure compliance with healthcare data regulations, and safeguarding patient confidentiality and trust. These features collectively enable the chatbot to provide personalized, effective, and secure healthcare support.
#GenerativeAI #PersonalizedMedicine #MedicalImaging #MachineLearning #ChatBots